Heavy Equipment IoT Takes Maintenance to the Next Level
- Blog
- Heavy Industry
- Heavy Equipment IoT Takes Maintenance to the Next Level
- Nov 30, 2017
- Cody Lirette
Recent headlines from the Wall Street Journal (The Big-Data Future Has Arrived) and Washington Post (Analytics puts midmarket companies in the big leagues) have illustrated that big data and analytics are in the mainstream. This is all pretty heady stuff, but what does it mean for the heavy equipment OEM, fleet owner, rental company or line manager.
Today, it’s a given that heavy equipment is equipped with telematics devices that provide detailed machine data. This data includes engine oil, transmission and brake temperature, engine RPM, speed, tire pressure, fuel consumption, emission levels among others. Depending on the manufacturer and telematics provider, more or less data is available.
Predictive Analytics is the practice of extracting valuable information from this data to determine patterns and predict future outcomes and trends. Predictive analytics does not predict the future but forecasts what might happen with an acceptable level of certainty. This technology learns from experience to predict the future behavior of assets to drive better decisions.
Consider traditional ways that vehicles are maintained. Fleets are put on a time-based preventative maintenance program where the oil is changed and parts are replaced based on elapsed time or mileage/hours used. This works well for normal wear and tear but does not help with random failures.
Reactive maintenance occurs when a random failure happens and a vehicle must be taken out of service. This is expensive both in terms of the cost of repair and the costs associated with unplanned removal of the vehicle from service, resulting in project delays and re-scheduling of downstream resources. A recent analysis showed that 80% of failures are random and not time or age based. Clearly, there is a need for a better maintenance methodology.
Predictive Maintenance, based on predictive analytics, detects possible failures ahead of time to take corrective action at the right time, to avoid unscheduled maintenance and unplanned downtime, thus mitigating project risks and reducing costs. A predictive maintenance solution is based on analysing machine data, historical failure data, and deep industry knowledge to predict an upcoming failure so that planned maintenance can be performed. This avoids the cost and delays associated with a random failure.
While this is a significant improvement over traditional maintenance approaches, there is more that can be done. Consider other sources of data that might improve analysis and add additional dimensions to decision making.
Operational data describes how an organization functions daily. In the context of a construction company, this might include machine operator schedule and history, technician maintenance assignments, or to which project part of the fleet is assigned. Analyzing this data provides insight into how, when, and by whom a machine is being used and maintained. Another source of data—business data—might include capital costs and depreciation, machine warranty, machine maintenance history, and spare parts inventory.
Combining, storing, and analyzing all this data is the kind of ‘deep learning’ required to take predictive maintenance to the next level. There are many important benefits of this approach. First is that we go from predicting when a known failure mode might occur and then acting to prevent it, to predicting and preventing a previously unknown type of failure. This new learning is then incorporated to improve domain knowledge which in turn helps build better models. This virtuous cycle continues as more data and data sources are incorporated and analyzed. Taking this to the next level, as we better understand failure modes and their predictors, and collect even more rich data on a per machine basis, we can model behavior for individual machines. These individual predicative models are important since machines of the same type are operated by different people and are used in different conditions. By having individual predictive models, we get to the holy grail of predictive maintenance that can allow us to reduce machine downtime by up to 50%.
Equally important are some non-maintenance related benefits. Combining telematics data with logistics data could allow for greater insight into drivers who are particularly tough on their machines, flagging the need for driver coaching. It could flag a technician whose machines have less problems in the field, providing an opportunity to share best practices. For heavy equipment OEMs, this data could facilitate better design and performance of their machines. Bringing business data into the analysis could result in a decision not to spend $40,000 on parts when a machine is coming towards the end of its useful life.
The technology for aggregating and analyzing this big data exists today. Applying analytics to this aggregated data is where new and important insights can be found and where business outcomes can be improved. The challenge for organizations is to share the data between departmental silos and, perhaps more importantly, sharing the data between organizations.
From better maintenance outcomes, to improved asset lifecycle management to better machine design, the insights gained when data is shared benefits the entire ecosystem, from OEMs, to leasing companies, to equipment owners. Businesses that adopt solutions that enable predictive maintenance have an opportunity to improve businesses processes, reduce costs and increase competitiveness.
What other opportunities do you think big data and analytics make possible for heavy equipment fleets? Please share your thoughts in the comments below…
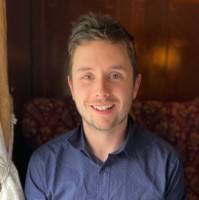
Cody Lirette is Senior Content Marketing Manager at ORBCOMM. With over a decade of marketing and communications experience for both the public and private sector, he uses his passion for innovative technology and plain language to build compelling content that inspires action.
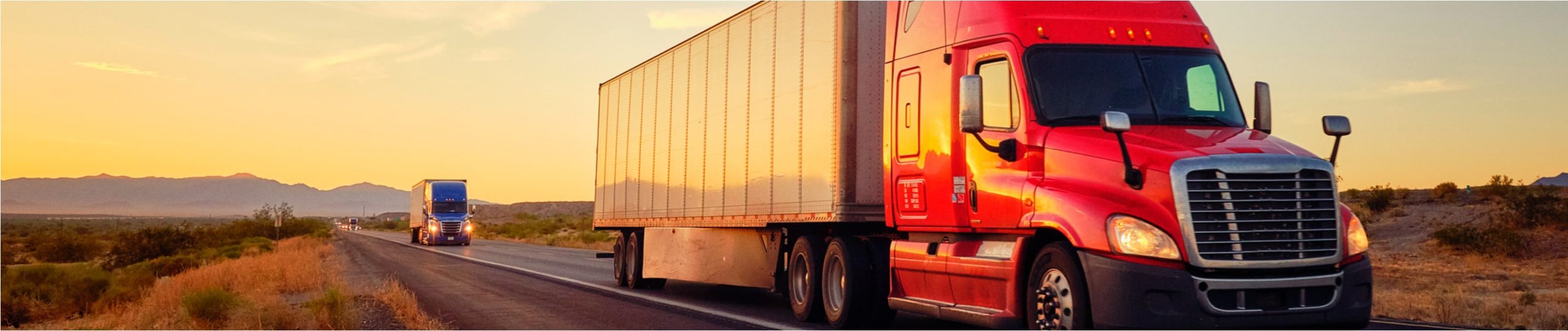